How AI in medical imaging is leading the way to better patient care
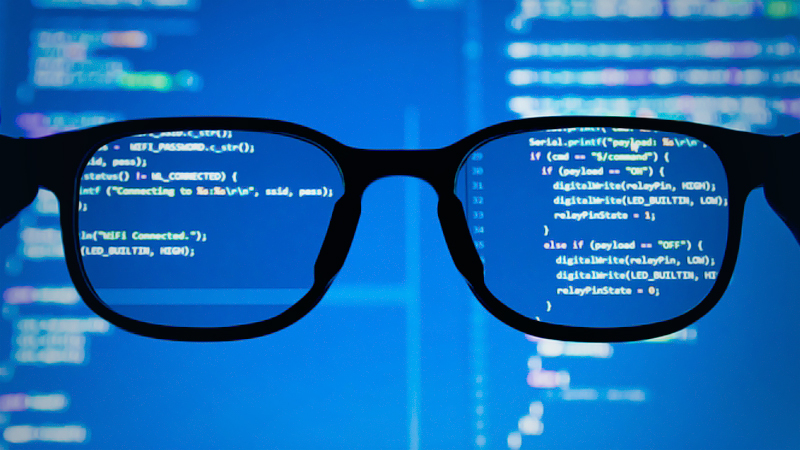
Artificial intelligence (AI) is now transforming key areas of healthcare, helping to save lives and reducing costs across the medical ecosystem.
One of AI’s most promising applications is medical imaging. This should come as no surprise, since radiologists have always pioneered digital technology in medicine.
As a result, the use of AI in medical imaging over the last 10 years has grown faster than in other specialties, according to the US National Institute of Health.
AI is now used frequently in magnetic resonance imaging and computed tomography. Other uses include interventional radiology, triage, aided reporting, follow-up planning, and infrastructure planning and prediction.
Pressure from ageing populations
Medical imaging is under pressure. Patient populations are ageing, which means the volume, complexity, and resolution of images are increasing, but the size of the radiology workforce is flat or shrinking.
In the developing world, the lack of radiology expertise is widespread.
Therefore, radiology departments need to carry out more examinations with fewer personnel. AI is a precious medical tool, enabling departments to make better use of limited resources.
By providing pre-screening or pre-analysis, AI operates as a co-pilot, helping radiologists be more efficient by pinpointing critical results – or exceptions – and enabling radiology teams to focus attention on patients who need urgent care first.
Earlier disease detection
Machine learning is being applied in all areas of medical imaging – from image acquisition to analysis to reporting.
For example, a medical start-up company is developing a suite of medical imaging applications that can enable contrast reduction, up to 4x faster scans, or both. This improves patient comfort and safety while increasing the productivity of a radiology department.
Deep learning models are being developed for a wide range of conditions, promising to increase the speed and accuracy of analysis and enable earlier disease detection.
High-profile areas under study include detection of lung nodules, brain cancer, multiple sclerosis (MS), breast cancer, and prostate cancer.
Some AI-powered diagnostic techniques are even moving beyond the clinic.
The University of Michigan Kellogg Eye Center is combining a smartphone-mounted device for retinal imaging with an AI software platform called EyeArt. This solution can determine in real time whether a diabetes patient should see an ophthalmologist for follow-up care.
Regardless of the AI application, usually the first step is collecting a data set with examples of both diseased/damaged and healthy tissue for the target condition. The data set must be prepared and, in most cases, clearly annotated. Today, annotation is still usually a time-consuming manual process.
Much of the significant AI work to date has been accelerated through the use of publicly available, annotated data sets. For example, for lung nodule studies, the Lung Image Database Consortium image collection (LIDC-IDRI) provides a set of computed tomography (CT) scans with annotated lesions. This data set was used for the Lung Nodule Analysis 2016 (LUNA16) challenge contest which concluded in early 2018 with significant results.
The big benefits
Accenture estimates that AI applications in US healthcare alone could save up to $150 billion (Bt4.7 trillion) annually by 2026. In cash-strapped community hospitals, those kinds of savings can help keep the doors open. In addition, AI has the potential to replace many of the menial tasks currently performed by radiologists, as well as integrating data mining into the electronic medical records process.
The positive, immediate impacts of AI in medical imaging can be numerous:
• Faster reporting with AI prepopulated reports that radiologists can edit for accuracy.
• Easier cohorting of studies for image or patient similarity.
• Better identification of studies with no significant findings. Many people assume AI is only good at finding abnormalities but what is proving more useful is the faster classification of normal or negative studies. This leaves the radiologist more time to review the abnormal ones.
• Better processing of electronic medical records, presenting the radiologists with timely, relevant clinical information about their patients.
• Built-in mechanisms for quality control and communication between radiologists and technologists.
AI is already impacting radiology, and more quickly than other medical fields. Any uneasiness among radiologists to embrace AI is analogous to how airline pilots were reluctant to embrace early autopilot technology. Obviously, AI is not going to replace radiologists. Instead, AI is going to help radiologists and the healthcare system in general.
Radiologists should be aware of the basic principles of how AI observations are obtained and how they should be interpreted. Datasets used to train AI models do have limitations and can potentially include bias. In this new paradigm, radiologists will need to know how to interact with AI solutions, how to flag studies that provided incorrect results or failed AI processing, and how to interact with the data scientists and IT supporting these solutions.
To this end, NetApp and NVIDIA are partnering to deliver the right AI solutions for the healthcare industry. Both companies are laser-focused on eliminating AI bottlenecks and advancing the realm of the possible at a rapid pace. NetApp’s attention to the data pipeline amplifies NVIDIA’s efforts to accelerate compute.
By combining technologies from both companies, NetApp’s ONTAP AI accelerates all facets of AI training and inference, to deliver better outcomes more quickly. The solution brings together NVIDIA DGX supercomputers, NetApp cloud-connected all-flash storage, and Cisco Nexus switches. This proven architecture simplifies, integrates, and accelerates both machine learning and deep learning algorithms, and allows you to start small and grow as needed without disruption.
The ONTAP AI Toolkit offers an array of tools and functions to simplify setup and operation, delivering immediate productivity.
Weera Areeratanasak is regional director of NetApp in Malaysia, Indonesia and Thailand.